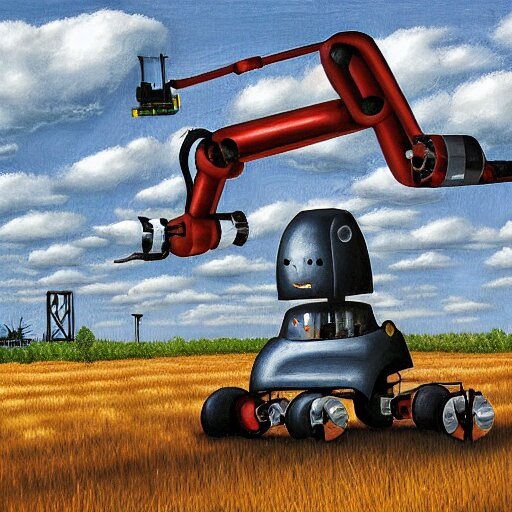
Digital Agriculture:
The building and controlling of the self-driving farm
The building and controlling of the self-driving farm
BY JIM THOMAS
The stages of digital agriculture and the power of the digital twin
To understand digital agriculture, first, we need to have a sense of a modern farm. Think of a modern industrial farm as a car. The farmer who “owns” or leases the car/farm is the driver. Using their knowledge of the road (the land) and of the car itself, the driver deploys techniques and technologies. For the driver, this might be the mechanics of a car, like an accelerator, power steering, and brakes. For a farmer, deploying technologies can mean nutrient management, irrigation, spraying etc. The farmer needs to manage inputs like fuel, repairs, chemicals, seeds, and fertilizer while navigating around hazards like the weather, disease, and market conditions. The destination is producing food for sale.
Now think of digital agriculture as steps towards building a self-driving car/farm. We can trace the transformation of digital agriculture through stages. Using the same analogy of a car, the farmer’s tech and control over the farm is radically changing every day. Below are the three stages so far of digital agriculture:
Now think of digital agriculture as steps towards building a self-driving car/farm. We can trace the transformation of digital agriculture through stages. Using the same analogy of a car, the farmer’s tech and control over the farm is radically changing every day. Below are the three stages so far of digital agriculture:
Digital Ag 1.0 - Digitally Informed
In the first stage, the driver uses sensors and information streams that tell them more about the road. They receive weather and traffic reports and alerts for hazards. The farmer, likewise, has tools that are equipped with sensors that monitor and feed them information about the land in real-time.
Digital Ag 2.0 - Digitally Nudged
At the second stage, artificial intelligence agents in the cloud monitor all of those streams of data to calculate better driving strategies. Beyond just displaying traffic data, an onboard digital advisor nudges the driver possible new routes or, in the case of farmers, agricultural strategies. To engage the farmer, the digital advisor might gamify this advice — offering discounts on farm inputs or other small digital rewards and ‘nudges’ to encourage (or manipulate) the farmer-driver to follow digital prescriptions.
Digital Ag 3.0 - Automated Driving
At a further stage, digital agriculture looks more like Tesla’s self-driving “autopilot “system” as automation increases. With fuller automation, the components are all determined and carried out by decision-making algorithms in the cloud — automatic chemical spray, seed drilling, harvesting, and irrigation, for example. These algorithms are informed and course correct in real-time by sensors and other situational data. The farmer may still believe they are “driving" the car because they ostensibly set the destination — but the minute-to-minute decisions on the self-driving farm are handled elsewhere.
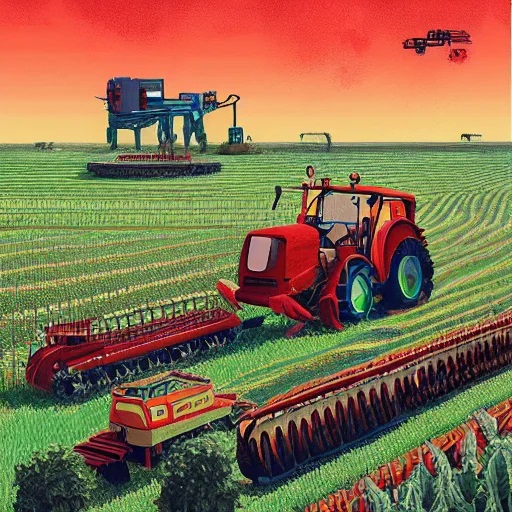
Establishing Digital Cybernetic Control Loops
In the example above, the move from farmer-determined production to an algorithmic-determined production is based on changing the “control loop,” as cybernetic designers call it. Cybernetics is the study of self-regulating systems, and a control loop is the flow of how a system self-regulates itself.
Control loops involve sensing, analysis, decision-making, and actuating (carrying out actions) — all coordinated in a continuous feedback loop that brings the system into regulation.
As digital and automated tools are introduced into this loop the farmer’s role in the self-regulation of the system first shrinks to just the analysis and decision-making part of the loop. Then that, too, is replaced on a day-to-day level by algorithmic analysis and decsion making in the cloud. Bayer-Monsanto estimate that there are 40 key farming decisions that north American commercial farmers need to make over a crop’s lifecycle. With their digital platform and digitally generated prescriptions, they offer that artificial intelligence can take over from the farmer the task of making those dozens of decisions. Their offer to busy farmers is that they will establish a digital control loop over individual farms — so that operations become increasingly efficient, self-driving, and require less human attention.
Machine Learning and Digital Twinging
This attempt to establish self-driving cybernetic control loops over complex economic activities is not limited to digital farming. The press tends to fixate on automation hardware (robotics, drones, etc.), but what makes control loops effective is the ability to carry out automated probabilistic decision-making in large racks of anonymous servers far away from the farm itself. This is known as Machine Learning (ML), which is the form of artificial intelligence now transforming many industries in the so-called ‘fourth industrial revolution.’
Machine learning systems are computer circuits that calculate the most probable answer based on what is already known. They work by gathering large amounts of data and then organizing that data to find relationships and calculate probabilities. In machine learning, the computer builds its own digital model of the object it collects data from. In the case of digital agriculture, the machine learning agents are compiling a virtual model of the farm and exploring the relationships between different variables reported by sensors (soil, climate, humidity, plant growth, prices, etc.) to make better probabilistic bets.
As more data is gathered and added, the model becomes richer and more detailed so the digital representation of the farm can be considered a useful copy or “digital twin” of the actual farm. Computing techniques can then be used to virtually manipulate this model digital twin to see how different types of interventions might change the real farm.
Michael Collins, who was formerly the strategic architect of Bayer’s digital platform, explains that “The essence of digital farming is the digital twin. The digital twin is the predictive production environment.” He explains that this digital twin “collects, stores, processes, and analyzes the sensed and non-sense information in the decision loops of the cultivation. It includes all aspects of the cultivation, including seed selection, soil management, stress determinations, and mitigation and harvesting. It extends into the farm management system to provide revenue and cost information. This model truly represents a digital twin of the farm and the current cultivation. This model, unlike its real-world equivalent, can be run many, many times to examine alternatives. It can be used to predict outcomes using data from previous outcomes and predictive results.”
This is how a digital farming platform, like Bayers Climate Fieldview generates farming prescriptions tailored to individual farms. When those prescriptions are enacted — especially by automated farm machinery — they can then be surveilled and course-corrected as real-time data confirms whether the predictions of the digital twin model are working out or not. In a feedback loop, the digital twin is adjusted and made more in line with the real-world farm. In a perfect loop, the automated farm itself would be efficiently nudged and course-corrected to fit the model — like the guided missile closing in on its target.
Using a ‘digital twin’ to drive real-world decisions and nudge outcomes is common in digital web platforms. Firms such as Facebook or Alphabet (Google) have built millions of digital twins of their users based on the constant stream of data that comes from smartphones, online activities, shopping data, etc. Critics of this approach have described these corporate-held twins as “digital voodoo dolls” that are shaped to resemble their real-life twins and ultimately exist to better manipulate and control users, like the reductionist stereotype of a voodoo doll.
This begs an obvious question: if agri-digital giants are establishing models of real farms to self-drive them through control loops, how might the corporate giants want to use that manipulation mechanism to their own ends?
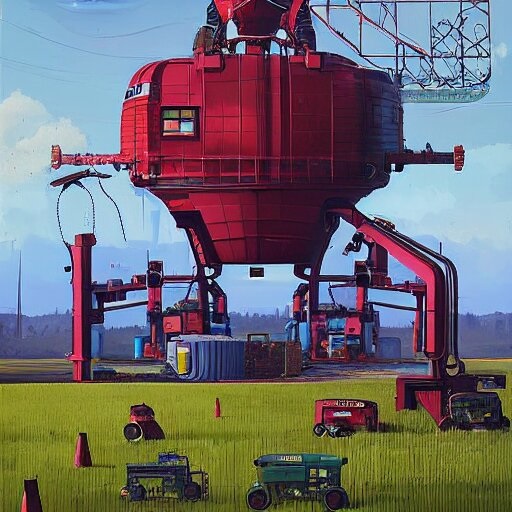
Beyond The Farm And Into The Entire Food Chain
One of the most notorious uses of digital twins to manipulate individual behavior was the Cambridge Analytica scandal, where an artificial intelligence-driven big data firm microtargeted millions of voters of whom they had built psychometric models to shift their voting behavior. In her book ‘Targetted,’ Cambridge Analytica whistleblower Brittany Kaiser reveals that the firm was also working with major food companies such as AB Inbev and Coca-Cola. Amazon Web Service — the cloud services arm of the world’s best known online store — actually sells ‘Twin Maker’ software as a service for companies to build digital twins and have themselves long used a digital twin approach to track (and nudge) their own consumers.
Although digital agriculture platforms supposedly build digital twins of the farm itself to generate prescriptions, one can expect they are also simultaneously building digital voodoo dolls of the farmers who use those systems to nudge, manipulate behavior and sell to them better. As with Cambridge Analytica, farmers may not be aware of the behavioral manipulation strategies practiced on them as digital consumers.
Casting a control loop over agriculture and the food chain is only one of the benefits of digitizing the food chain for agrifood giants, tech titans, and other data players. A large amount of data acquired from digital activities in one part of the food systems can also be applied to inform data models on other parts of the chain. For example, aggregating real-time crop production data across many geographies, when combined with weather data, economic prices and historical data etc. can generate digital twins of commodity markets to allow predictve financial speculation, hedging, and power investment plays. Combining food, health, dietary and consumption data could provide valuable insights into pharmaceutical strategies. As such the data streams from digital agrifood, especially when aggregated, analyzed and processed, become a valuable new commodity to sell or trade with other industrial sectors.
JIM THOMAS is an activist, writer, researcher and poet. I have almost three decades international experience tracking emerging technologies, ecological change, biodiversity and food systems - on behalf of movements and in UN fora. Until recently I shared in the work of the ETC Collective where I was formerly Co-Executive director and Research Director.
More on Jim ︎︎︎
More on Jim ︎︎︎